Seminar: Better quantifying and handling parametric uncertainty in water infrastructure design
Join the ANU Institute for Water Futures for a seminar by Julianne Quinn.
Speakers
Content navigation
Description
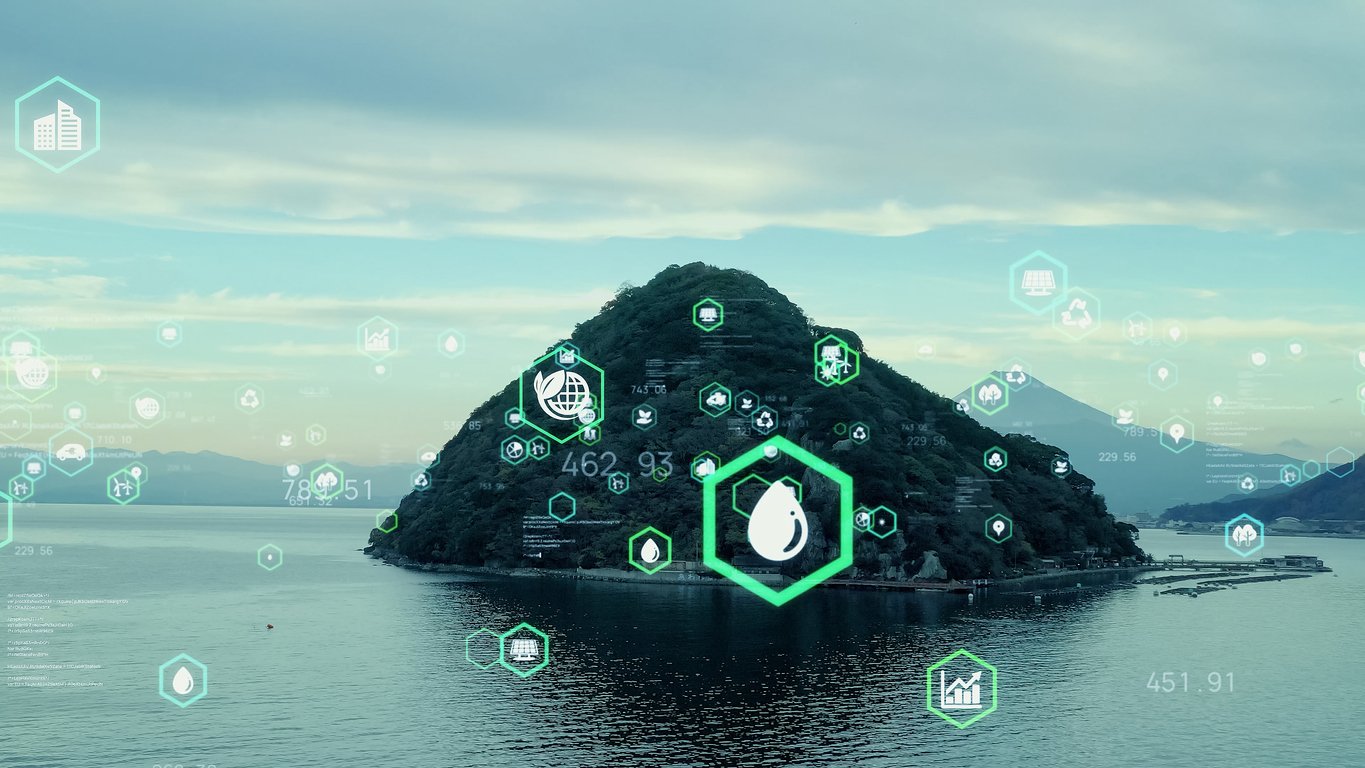
Join the ANU Institute for Water Futures for a seminar by Julianne Quinn.
Julianne is an Assistant Professor at the Departments of Civil & Environmental Engineering and Systems & Information Engineering at the University of Virginia. Her talk will focus on two major research questions related to the problem of quantifying and handling parametric uncertainty in water infrastructure design.
The seminar will be followed by a complimentary light lunch.
About the Topic
Stormwater infrastructure is an effective tool for combating the negative impacts of urbanization on flooding and pollution. However, the optimal location and size of such infrastructure needed to achieve these benefits depends on the spatial distribution of runoff and pollutant loads throughout the watershed. Unfortunately, limited sensing data prevents full spatiotemporal knowledge of these variables, requiring their estimation through hydrologic models whose parameters are highly uncertain. Yet design approaches that account for this uncertainty are rare. This is concerning, as designing to a single assumed parameter set could lead to significant over or under-investment. At the same time, quantifying parametric uncertainty via Bayesian inference can be computationally expensive, limiting its use in design.
This talk will focus on two research questions related to the problem of quantifying and handling parametric uncertainty in water infrastructure design: 1) “what Bayesian estimation algorithms are most effective on high-dimensional, multi-modal inverse problems like hydrological model calibration to make designing for uncertainty computationally tractable?” and 2) “after quantifying parametric model uncertainty, how should we best account for this uncertainty in designing infrastructure investments to balance socio-ecological tradeoffs of urbanization and reforestation?” The first part of the talk will discuss a new set of diagnostics our group has developed to quantify the efficiency (speed), reliability (consistency) and controllability (insensitivity to algorithm hyperparameters) of different Markov Chain Monte Carlo (MCMC) algorithms in estimating the true posterior distribution of complex inverse problems. The second part of the talk will compare alternative robust optimization approaches for handling hydrological model parameter uncertainty quantified by MCMC algorithms using a multi-objective reforestation problem. These studies provide insights into how to make quantifying and designing for parametric uncertainty in hydrological models computationally tractable, improving water infrastructure design.
About the Speaker
Julianne Quinn is an Assistant Professor in the Departments of Civil & Environmental Engineering and Systems & Information Engineering at the University of Virginia. Her research focuses on advancing optimization and simulation methods used to inform the design and management of water resources systems with the goals of protecting people from nature (floods and droughts), and nature from people (pollution and consumption). She treats this as an iterative process between risk and uncertainty analysis to discover which sectors and populations are most vulnerable to natural and anthropogenic hazards and multi-objective optimization of the system to reduce these multi-sectoral vulnerabilities. She is particularly interested in how advanced sensing and forecasting techniques can be used to inform this optimization. She received her B.S. in Earth and Environmental Engineering from Columbia University in 2011 and her Ph.D. in Civil and Environmental Engineering from Cornell University in 2017.